Interview with first author Mara Steiger about her study on Neurosurgery
In our interview series, we talk to the first authors of recent studies and take a look behind the scenes of science
MPIMG doctoral candidate Mara Steiger is one of the first authors, together with Björn Brändl (CAU, Kiel), of a recent joint paper in Nature Medicine from the Kretzmer lab (formerly at the MPIMG, now at the HPI, Potsdam) and the group of Franz-Josef Müller at the UKSH in Kiel. We talked with her about her motivation to pick up this project, what she enjoyed most about the work and how it helped her to grow professionally.
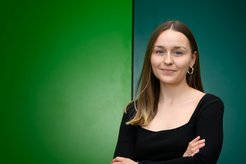
Mara, can you summarize the findings of the paper, with a focus on your part?
We developed a machine learning model, based on a classical statistical theorem, that uses sparse methylation data to characterize the epigenome of tumors. With our collaborators, we used this approach to create a framework that allows for ultra-rapid classification of brain tumors during surgery using Nanopore sequencing. By delivering actionable insights in under one hour, this approach has the potential to help surgeons adjust their strategy in real time and may improve patient outcomes.
What was your motivation to start the project?
I joined the project at a later stage through the IMPRS-BAC graduate school and it was actually a perfect fit with my previous experience. I have a background in bioinformatics with a focus on data science and a particular interest in genome regulation. For my undergraduate degree, I worked here at the MPIMG with Martin Vingron on predicting enhancer activity. For my master's thesis I went to the FLI in Jena where I did a project on age prediction based on DNA hydroxymethylation. That's why I was immediately interested in this project, as it felt like a natural extension of my previous work, which similarly focused on epigenetics and the application of machine learning in this context. What made the project even more interesting to me was the opportunity to apply these methods in cancer diagnostics.
What was the biggest challenge in the project?
One of the biggest challenges – but also a huge opportunity – in such long-term, collaborative projects is working in a cross-disciplinary team. Effective communication between partners, with expertise ranging from wet lab and surgical oncology to bioinformatics, was essential. The requirements, approaches, and workflows often differ greatly, so we had to develop a mutual understanding of each other’s methodologies and perspectives to bring them together in a meaningful way.
What kind of new skills did you learn?
Definitely this interdisciplinary communication. But I have also learned to keep track of everything in a project while occasionally stepping back to zoom out and look at the project as a whole. It's important not to get too caught up in small details and to always keep the bigger picture in mind. I would also say that I picked up a lot of new methodological skills.
What was most rewarding for you?
I really enjoyed optimizing the statistical model. The initial implementation worked quite well, but especially in my first year here, I focused on fine-tuning the details, which significantly improved the performance of our model.
What was also very interesting to see is how third-generation sequencing technologies open up completely new possibilities in the clinic – such as enabling rapid epigenome-based tumor classification – that simply didn't exist before. But these advancements also bring new challenges and require unique approaches. That’s why it was super exciting to discover that a relatively simple yet carefully optimized statistical model was ideal for addressing these challenges, especially given the limited data availability.