Scientific projects
Omics analyses in cancer research
Some of our recent projects are described below.
A) Epigenomics of non-small cell lung cancer

Figure 1: Response DMRs in gene promoters that correlate with tumor volume of PDXs after therapy.
Non-Small Cell Lung Cancer (NSCLC) is primarily treated with radiation, surgery and platinum-based drugs like carboplatin and the major challenge is intrinsic or acquired resistance to chemotherapy. Molecular markers predicting the outcome for the patients are urgently needed. In the BMBF-funded project EPITREAT we employed patient-derived xenografts (PDX) to detect predictive methylation biomarkers for platin-based therapies (Grasse et al., 2018). Using MeDIP-seq we generated genome-wide DNA methylation profiles of PDXs and identified a set of candidate regions with methylation correlated to carboplatin response and corresponding inverse gene expression pattern even before therapy (Fig. 1). This analysis led to the identification of a promoter CpG island methylation of LDL Receptor Related Protein 12 (LRP12) associated with increased resistance to carboplatin. Validation in an independent patient cohort confirmed that LRP12 methylation status is predictive for therapeutic response of NSCLC patients to platin therapy with a sensitivity of 80% and specificity of 84% (p < 0.01). Additionally, we find a shorter survival time for patients with LRP12 hypermethylation in a validation cohort (Fig. 2) as well as in the TCGA cohort for NSCLC (LUAD; Fig. 3) confirming the results.

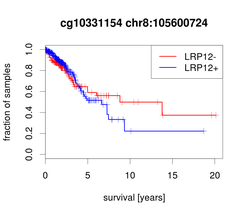
B) Genetic predisposition in colon cancer
We have applied our previously developed workflows for RNA-seq and MeDIP-seq (Lienhard et al., 2017) to further project collaborations in the field of colon cancer. In particular, we were interested in the question how genetic predisposition can influence the penetrance of tumor-initiating mutations. With the Herrmann department, we have conducted a fundamental cancer genetics study based on consomic mouse strains to identify modifiers of intestinal tumor formation. We have identified candidate gene regions and biomarkers on mouse chromosome 5 that determine tumor multiplicity in the presence of cancerous APC mutations (Fig. 4; Farrall et al., 2021).

A novel framework for network genome analysis
The Herwig group has contributed resources and methods for the network-based analysis of genome data. Since 2009 we continuously provide the ConsensusPathDB resource, a meta-database of human (as well as mouse and yeast) molecular interactions integrated from 33 public resources.
This database has recently been substantially updated with content and novel interaction types and currently comprises 859,848 interactions of different types for 200,499 different physical entities such as proteins, drugs, metabolites etc. (Kamburov and Herwig, 2022). In particular, the integrated protein-protein interaction (PPI) network has been used as a scaffold for genome analysis, and we have developed a mathematical network propagation framework, called NetCore, based on random walk with restart to analyze experimental data from multiple sources at the network level (Fig. 5). Instead of node degree, the algorithm utilizes node core for the re-ranking procedure twhat is specifically robust against experimental bias of PPI experiments (Barel and Herwig, 2020).
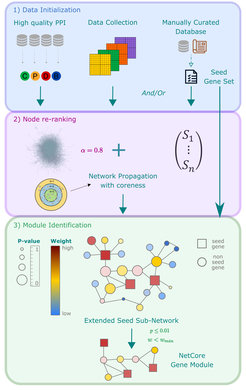
Such network propagation has been applied in the context of multi-omics drug toxicity, exploring toxicity mechanisms and clinically relevant novel biomarkers (Fig. 6A-B; Selevsek et al., 2020).
Ongoing projects include the network-based analysis of phosphorylation data to study the temporal response to insulin treatment in human muscle in cooperation with the German Diabetes Center in Düsseldorf as well as the computation of network-derived drug safety indices for the improvement of drug development in a joint BMBF-funded project with the University of Maastricht.

Enhancing biological plausibility for machine learning methods in biomedicine
The Herwig group participates in the AI initiative of the Federal Ministry of Education and Research (BMBF) and has coordinated a project to improve machine learning for biomedical applications by the incorporation of biological background knowledge and the exploitation of methods that enable post-hoc interpretability of machine learning methods.
A major application domain is the survival risk prediction for cancer patients with molecular data. We have developed an approach, “Predict and Propagate”, that is based on ensemble tree learning with XGBoost and subsequent network propagation of the learned features with NetCore that generates plausible network modules from otherwise non-interpretable machine learning methods (Fig. 7; Thedinga and Herwig, 2021, 2022). In particular, we found that the tumor microenvironment is highly predictive for pan-cancer patient survival.

A second application domain is the prediction of drug sensitivity from the molecular characterization of the cellular system and chemical descriptions of the drug under study. In cooperation with machine learning experts at the University of Potsdam, we developed a method based on the minimization of ranking loss for drug sensitivity predictions (Prasse et al., 2022a), which outperforms existing deep neural network approaches. Currently, we work on transfer learning protocols and explore deep neural network architectures. This allows us to use the large body of in vitro drug sensitivity data for pre-training and subsequently use these pre-trained models for the prediction of drug sensitivity in pre-clinical systems, such as PDXs, PDOs and ex-vivo patient tissues, where training data is typically of much smaller size (Prasse et al., 2022b). With the developed methods we have contributed to two international machine learning challenges, the BEAT-AML challenge on drug sensitivity predictions for AML patients and the IDG-DREAM challenge on binding properties of kinase inhibitors (Cichonska et al., 2021).
Long-read transcriptome sequencing (LRTS)
A new development of the group is the investigation of alternative splicing using 3rd generation sequencing techniques, in particular long-read RNA sequencing. This technology allows unprecedented insights into gene isoform structure since long sequence reads capture long-distance splicing events and complete isoforms.
The Herwig group has developed the software package IsoTools, a complete pipeline for mapping, annotation and statistical analysis of PacBio Iso-Seq® experiments. The package comprises data quality control steps, isoform identification and quantification, identification of (coordinated) splicing events, as well as statistical tests for differential splicing (Lienhard et al., 2021).

In a project funded by the DFG we, in cooperation with groups at the Universities in Cologne and Frankfurt, applied IsoTools to investigate aberrant splicing induced by mutations in the splicing factor SF3B1 in chronic lymphocytic leukemia (CLL) patients and patients with myelodysplastic syndromes (MDS) (Pacholewska et al.). We observed that SF3B1 hot-spot mutations specifically affect 3’ alternative splicing (Fig. 8A) and, using iCLIP data, we identified an RNA binding signature that suggests a double peak at -50 and -10 nt upstream of the 3’ splice site (Fig. 8B). This might have effects on the binding capabilities of SF3B1 as well as on branchpoint and 3’ splice site recognition. Ongoing work includes the experimental validation of these findings.
Additionally, with IsoTools we participated in LRGASP, an international challenge on long-read transcriptome isoform identification and quantification organized by the Gencode consortium. Dissemination is strengthened through joint tutorial session at ECCB 2022.
Future projects will cover the extension of the analysis framework to single-cell protocols and the development of refined patient-specific isoform-landscapes. This will be realized by the involvement of the group in the European-wide Marie Sklodowska-Curie action LongTREC on LRTS and its applications in biomedicine, starting in 2023.